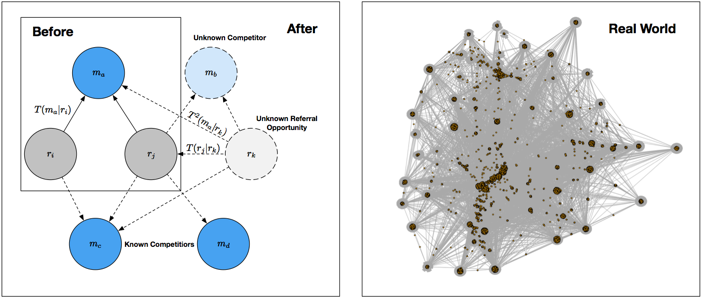
Many marketers struggle to identify and prioritize opportunities because they do not have the analytical tools to quantify the value of traffic sources within an evolving market ecosystem. Tools such as Google Analytics are fantastic for obtaining granular insight into the performance of existing sources of referral traffic, but in isolation leaves one blind to the context and value of those inbound traffic sources within the broader competitive landscape.
At DemandJump, we apply graph theoretic algorithms to inbound traffic data for all domains within our users’ competitive ecosystems to identify top opportunities for our users.
For the uninitiated, graph theory is the study of patterns that arise from the relationships between sets of vertices V and the edges E that connect them. In our model, we have two types of vertices: competitive market domains M and referral source domains R. Relationships between vertices are defined by a positive transfer of traffic T(M|R).
We consider not only the relationship between referral sources to market domains, but the relationship between referral sources. This structure forms a map of the market that expands out to include first, second, and third degree traffic connections to our users and their competitors.
All of this allows us to create a dynamic understanding of the referral ecosystem of our users and their competition.
The value of each traffic source in the network is computed in two parts: 1) the known direct traffic contribution of that source to our users and their competitors and 2) the estimated indirect contribution of traffic to our market through other referral sources.
By dramatically expanding the scope of our analysis to the full market, we are able to identify previously unknown referral opportunities for our users and even unknown competitors based upon the connectivity properties of each node in the expanded network and the two part value function.