What is Marketing Mix Modeling?
November 4, 2020 •DJ Team
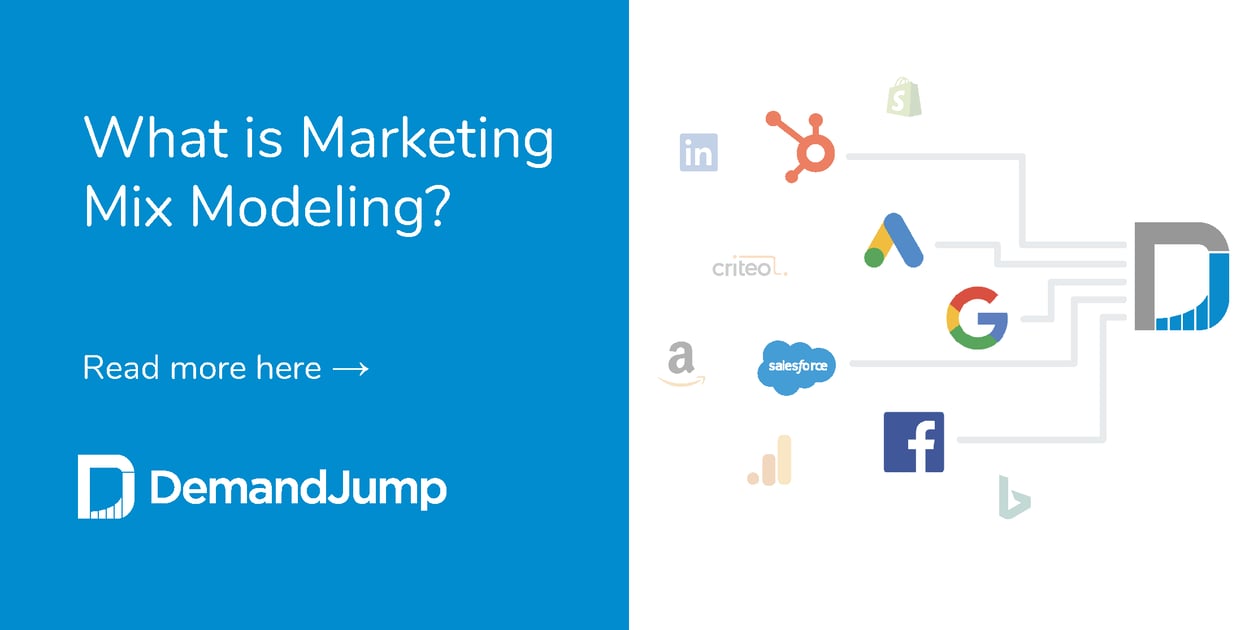
Marketing strategies are all about customer engagement, plain and simple. You’ve mastered your brand and spent months researching consumer needs and pain points, All that’s left now is to connect the dots and show people why and how you’re going to change their lives. But if marketing is intended to merge these two lanes - your business identity and potential customers - what’s the best way to determine if the process was successful?
This is where the concept of marketing mix modeling comes (also known as media mix modeling) into play. Utilizing advanced statistical analysis methods, marketing mix modeling (MMM) helps digital marketers to establish connections between their specific strategy and elements with tangible numbers like sales goals and customer retention. But in an even larger sense, MMM can help your marketing team define ROI.
How Does Marketing Mix Modeling work?
The main function of Market Mix Modeling is to translate the value of marketing efforts into a direct and demonstrable connection to something happening in sales, market share, and return on investment. To do so, the practice focuses on comparing aggregate historical data between marketing and sales metrics.
- The MMM approach is based on a popular marketing theory known as the 4Ps of the Marketing Mix, which are: product, price, place, and promotion. This theory states that these are the four fundamental elements of any successful business. The purpose of MMM, then, is to measure how much success came from each of these elements, and create projections for maximizing success through optimization of the mix.
- In order to do so, MMM compares aggregate data to understand, identify, and differentiate which factors led to the specific success. Was it marketing efforts and promotions? Or was there some external factor at play?
Throughout the process, marketing mix examples rely on the use of multi-linear regression to identify a correlation between an independent variable x and a dependent variable y, where the value of y can be predicted by measuring x.
Dependent variables represent the hard, financial data that illustrates success. This might mean sales, market share, or stock price.
The independent variable represents the marketing efforts. These are broken into two categories; Above the line (ATL) and Below the line (BTL). ATL activities would include print, radio, TV, and digital ads. BTL activities are temporary promotions, sales, coupons, contests, and direct mail marketing.
- From here, marketers form an equation between two variables. The line drawn from this equation could be linear or nonlinear, i.e. connected or not connected.
- For example, a TV or digital advertisement may have an effect on brand awareness, but it can’t have a linear relationship to an increase in sales; rather, we need to look to non-marketing factors. These are known as base drivers, and can include price, distribution trends, seasonality factors, and other macroeconomic influences.
The Benefits of Marketing Mix Modeling
By identifying and measuring the discrete factors that led to a specific instance of success, marketers can draw educated and informed conclusions. This makes it much easier to create blueprints for future growth, specifically by:
- Analyzing marketing spend and budgets to get the most out of every dollar. Consider the non-linear relationship between advertising and sales; most models show that while ad spend can increase awareness, there’s a limit to its efficacy. After a certain point, spending any more on ads could be a total waste of money; in another scenario, the push from ads may not come until after a certain dollar threshold. MMM helps to establish those price points
- Establish accurate forecasting for future marketing strategies. With a handle on which marketing efforts work best, as well as which ones are trending up (or down) teams can plan out their strategies further into the future.
- Uncovers hidden or ignored correlations that the company could lean into. For example, it may be hard to explain, but maybe people in Minnesota just like a car more than people in Detroit. You can’t change instinct, so why not create more marketing around those correlations?
In general, MMM provides high-level insights into marketing campaigns and strategy, as well as shedding light on the trends that could be most impactful.
How to Use Marketing Mixed Modeling
While these techniques are mathematically sound, there have also been claims that the MMM is a kind of dead marketing language, done away by time and more advanced tech tools. For instance, there’s the concern that MMM doesn’t provide enough insights on the consumer level, or help marketers to create customized messaging. Additionally, the use of historical data over the course of two or three years means infrequent reporting.
However, MMM can be greatly beneficial when it’s performed once or twice a year as part of a larger marketing strategy. This allows marketers to still benefit from those high-level insights, and then keep those in mind when using more granular data analysis techniques like data-driven or multi-touch attribution. MMM also provides insights into the kind of offline conversions that occur in-person with a sales team.
Why Marketing Mix Modeling Words Best with DemandJump
The truth is, MMM isn’t extinct or out-of-date, it just provides a different vantage point and perspective on marketing analysis. It may have been invented before Big Data was a buzzword, but now it can not only integrate with huge datasets, but can be accelerated by the use of cutting-edge technology. The data used in MMM is aggregate, meaning it’s made up of several years of metrics and numbers. This also requires clean data that is easy to integrate into modeling software. That’s where DemandJump comes in. Our platform allows you to get as in-depth as you’d like with marketing data, and you can alter those timelines to stretch as far back as you need. Ready to get started? Start your Free Trial Today!
Featured Articles
Categories
- Attribution Tracking (13)
- Channel Optimization (11)
- Consumer Insights (68)
- Content Marketing (251)
- Data Science (8)
- Digital Marketing (6)
- Digital Transformation (26)
- Enterprise (10)
- Lead Generation (14)
- Market Intelligence (8)
- Marketing Analytics (39)
- Marketing Attribution (57)
- Marketing Management (153)
- Marketing Operations (86)
- Organic Search (222)
- Paid Search (52)
- Pillar-Based Marketing (63)
- Programmatic Advertising (9)
- SaaS Content (14)
- SaaS Marketing (29)
- Search Marketing (111)
- SEO Keyword Research (28)
- SEO Pillar (18)
- SEO Strategy (46)
- SMB (5)
- Website Content (12)